Ethical Considerations in Data Science
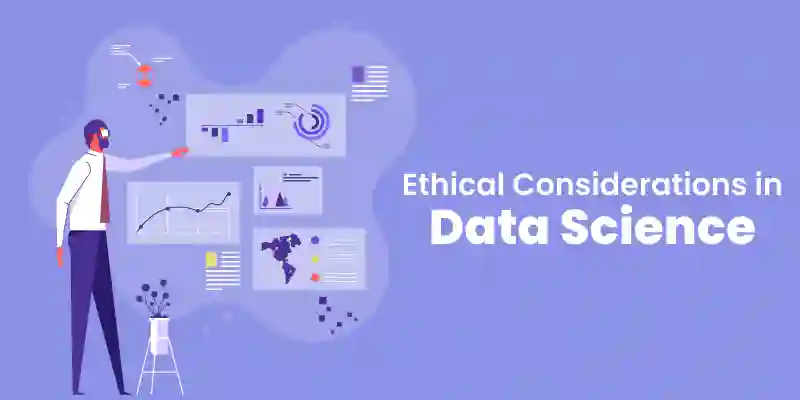
In the rapidly evolving field of data science, where the promise of innovation meets the responsibility to protect individuals and society, ethical considerations play a pivotal role. As we harness the power of data to derive meaningful insights, it becomes imperative to navigate this landscape with a keen awareness of the ethical implications involved.
Achieving a Data Scientist Certification enhances credibility and demonstrates a commitment to ethical practices. It validates expertise, instilling trust in stakeholders and showcasing the ability to navigate complex ethical considerations in data science. Now, let’s understand the ethical considerations in data science, exploring the challenges and responsibilities that come with the ever-expanding realm of data.
Privacy Concerns
Privacy concerns in data science represent a critical ethical dimension, demanding careful consideration and proactive measures. As data scientists gather and study huge amounts of private data, there is great risk of misusing information and violating personal privacy. A key challenge is finding an delicate equilibrium between deriving useful knowledge from data analysis and upholding individuals’ rights to keep their personal details confidential.
Transparent practices become imperative in addressing privacy concerns. Data scientists must be explicit about the purpose and scope of data collection, ensuring that individuals are well-informed about how their information will be used. Obtaining informed consent emerges as a key ethical principle, empowering individuals to make autonomous decisions regarding the sharing of their data. This involves clearly communicating the intended use of data, potential risks, and the safeguards in place to protect privacy.
Bias and Fairness
The ethical consideration of Bias and Fairness revolves around the potential for algorithms and models to produce outcomes that systematically favor or disadvantage certain groups of individuals. Bias in data can manifest in various forms, stemming from historical inequalities, societal prejudices, or skewed data collection processes.
Data scientists need to be keenly cognizant of the likely prejudices within their data, since those biases could propagate and intensify current societal inequities. Regardless of whether it is gender, race, socioeconomic position, or other attributes, prejudiced algorithms can result in discriminatory consequences. For example, biased facial recognition algorithms may inaccurately identify people from certain ethnic groups more often than others.
Addressing bias requires a proactive approach. Data scientists should meticulously examine their datasets, identify potential sources of bias, and employ techniques to mitigate these issues. This includes using diverse and representative datasets, applying fairness-aware machine learning techniques, and regularly auditing models for unintended biases.
Transparency and Accountability
Transparency and accountability form the ethical bedrock of data science, ensuring that the decision-making processes and outcomes are clear, justifiable, and subject to scrutiny. Transparency involves providing a clear and comprehensive view into the methodologies, algorithms, and data sources employed in a given analysis or model. This transparency extends to the disclosure of potential biases, limitations, and uncertainties inherent in the data, fostering a culture of openness.
Accountability, on the other hand, demands that data scientists take responsibility for the consequences of their work. This involves documenting key decisions and rationale throughout the data science lifecycle. By maintaining a clear audit trail, data scientists enable stakeholders to trace and understand the logic behind algorithmic outputs. Accountability also implies responsiveness to challenges or discrepancies raised by external parties, fostering trust and credibility in the broader community.
Security Challenges
Security challenges in data science encompass a multifaceted landscape that requires vigilant attention to safeguard sensitive information. One primary concern is unauthorized access, where malicious actors attempt to breach systems and gain illicit entry to confidential data. Robust access controls and encryption protocols are imperative to thwart such attempts, ensuring that only authorized personnel can interact with and manipulate sensitive datasets.
Data integrity is another critical facet of security challenges. Ensuring that data remains unaltered and accurate throughout its lifecycle is essential for reliable analysis and decision-making. Data scientists must implement checksums, hashing techniques, and regular integrity checks to detect and rectify any tampering or corruption.
Additionally, cyber threats like ransomware and phishing attacks are becoming more common and pose a major risk to data security. Data scientists need to stay updated on emerging cybersecurity threats and take proactive steps to bolster defenses against potential intrusions. This includes regularly updating software, using firewalls, and implementing intrusion detection systems. Maintaining robust safeguards is essential as threats continue multiplying in scale and sophistication.
Informed Consent
Informed consent is a fundamental ethical practice that entails obtaining explicit and voluntary agreement from individuals prior to the collection, processing, or utilization of their personal data. It serves as a cornerstone principle that upholds individuals’ autonomy and enables them to make well-informed decisions regarding the handling of their information.
Obtaining informed consent necessitates data scientists to communicate information in a clear and transparent way. This involves explicating the rationale for data gathering, delineating the planned application of the data, and tackling any prospective hazards or ramifications linked to its processing. Moreover, the communication must be delivered in a language that is easily graspable and accessible to individuals, regardless of their technical adeptness.
Informed consent transcends a mere checkbox on a form; it constitutes an ongoing process characterized by continuous communication. Individuals should have the opportunity to withdraw their consent at any point. This principle holds particular significance in contexts involving sensitive or personal information, such as healthcare or behavioral analysis. Abiding by the principle of informed consent not only maintains ethical norms but also nurtures trust between data scientists and the people whose data they manage. This, subsequently, conduces to the emergence of a culture focused on accountable and respectful data utilization.
Social Impact and Responsibility
The ethical dimension extends beyond individual transactions and algorithms, encompassing the broader social impact of data-driven initiatives. Social impact and responsibility in data science entail a conscientious awareness of how analytical outcomes influence communities and society as a whole.
Data scientists shoulder the responsibility of understanding and mitigating potential negative consequences that may arise from their work. By recognizing the broader implications, practitioners can actively contribute to positive societal change. This involves using data to address pressing social issues such as healthcare disparities, educational inequalities, and environmental challenges.
Moreover, ethical data science prioritizes the inclusion and representation of diverse perspectives within datasets and analyses. Recognizing the potential for biases in data and algorithms, practitioners strive to create models that are fair and just. By doing so, they contribute to dismantling systemic disparities rather than perpetuating them.
Conclusion
Ethics matter in data science. Those handling lots of information, like data scientists, must ensure their work meets high ethical standards. By valuing privacy, tackling bias, being transparent, ensuring security, getting consent, and thinking about the wider impact, data scientists can handle ethical challenges. In the changing world of data science, sticking to ethical practices is crucial for using data to benefit people and society.